The Bank of the North - A Quick Case Study for HDFS Modernization
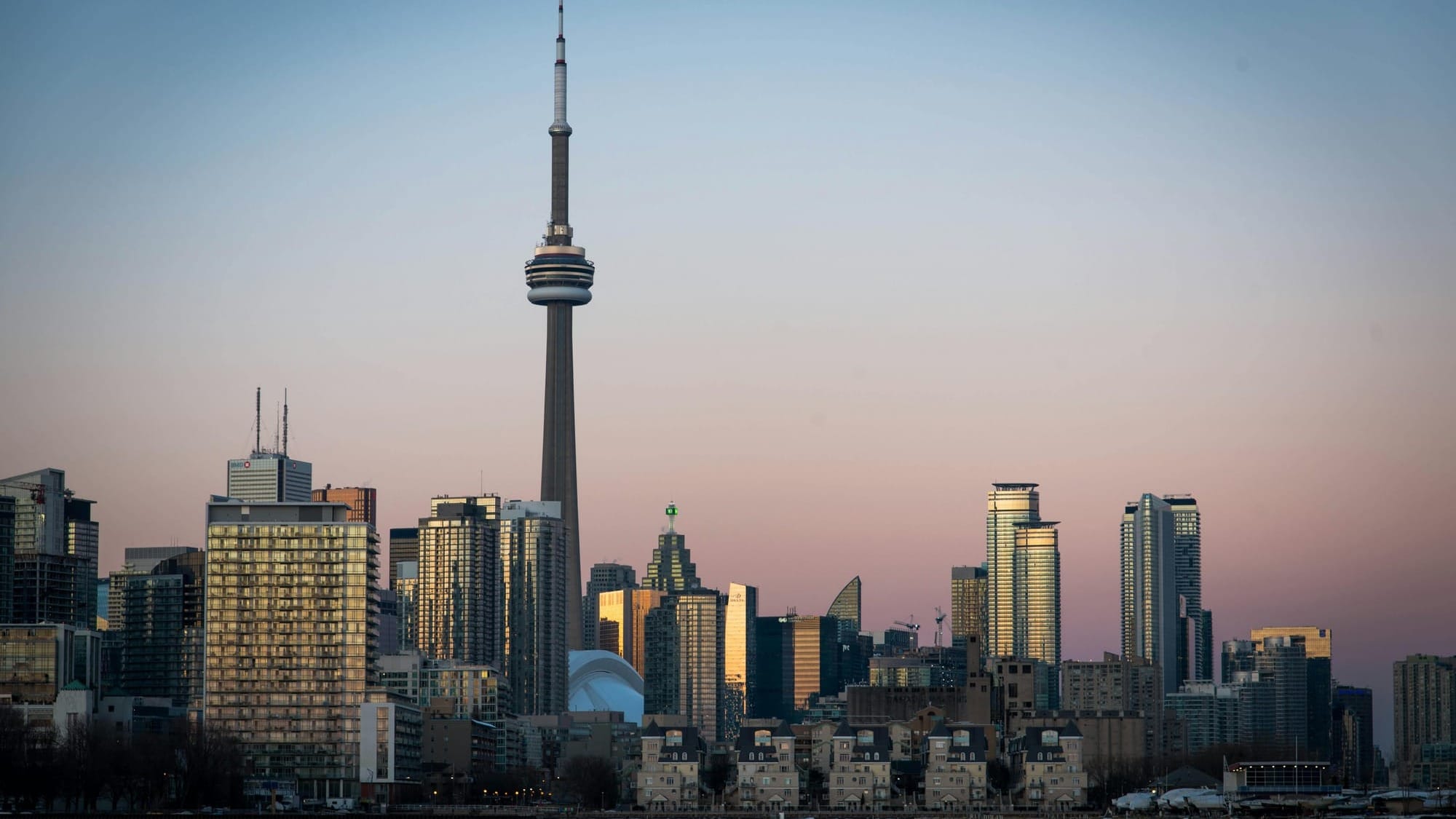
Stories matter and customer stories are the best. The ones where they delivered jaw-dropping stats or overcame massive obstacles are the ones that garner the best headlines. They are also the ones that are the hardest to get published. We know, because we are going to share a few with you that we are tirelessly working to get published - but for now they will remain anonymous. Having said that, if you want to contact us, we can let you look behind the curtain.
Let’s get started.
Bank of the North
We do business with all of the major Canadian banks. There aren’t that many that are truly global institutions so your chances of guessing correctly are pretty good. Like other major financial institutions, they have regulatory obligations to generate and store electronic records from every client interaction. Big data analytics enables these institutions to not only store data for regulatory purposes but also actively leverage this information to generate business insights and add value. Machine learning and artificial intelligence (ML/AI) technologies are driving modern data-intensive workloads to not only conduct historical data analytics but also perform real time analytics to drive immediate decision-making. Real time analytics use cases include fraud detection, trade surveillance, customer segmentation, personalized marketing and risk management.
This bank migrated their antiquated enterprise data warehouse (Cloudera/Hadoop) to MinIO when they started to experience performance and stability issues - as data grew within the Hadoop environment, applications started experiencing performance issues and downtime. They wanted the cloud operating model without the cost and loss of control associated with the public cloud. The challenges associated with data growth coupled with the need to modernize infrastructure provided a roadmap to a modern, cloud-native, Kubernetes-based architecture. The bank wanted a clean implementation and shortest time to market. That meant MinIO.
The storage infrastructure supports multiple business units including:
- Canadian Banking Analytics
- International Banking Analytics
- Data Enablement & Architecture
They run two MinIO deployments in separate data centers. Data between the two is replicated using active-active replication, allowing MinIO to be highly available. Given the critical nature of the bank’s financial data, an ironclad requirement was for the MinIO object storage implementation to support infinite scaling and site level disaster tolerance. To achieve this, the MinIO team helped deploy a dual site active-active replication strategy. The bank has the ability to grow the size of the cluster at each site on demand, by simply adding new server pools to the deployment. With active-active site level replication, not only can the bank’s MinIO implementation survive multiple disk, server and even rack failures within a single site, but the entire site within a given geographic location could experience a failure without applications any downtime or data lossFor example:
Cluster One:
Cluster Two:
One of the main use cases for on-prem object storage is Enterprise File Handling, where currently over a hundred projects have been migrated to take advantage of the MinIO object store. The bank leverages MinIO for machine learning model training and serving:
Scalability and Availability of the MinIO Cluster:
Synchronizing data between multiple data centers is a key capability of any object storage that provides for site-level disaster tolerance. Active-active replication provides for fast hot-hot failover and muti-geographic resiliency. Multi-site replication builds on the two-way active-active framework and retains key functionality, such as replication of delete operations, delete markers, existing objects and replica metadata changes.
Results
The benefits of upgrading to a modern, Kubernetes-based infrastructure were immediately apparent. Since implementing MinIO, the bank has cut their storage footprint by more than 50% while simultaneously doubling their storage capacity. In doing so, the bank reduced costs by nearly 60% and improved the performance of key machine learning tasks by 30%.
MinIO also positions the bank for future cloud-native success. The platform engineer stated that “Data needs that constantly evolve (such as ours) require scalability and robust storage location, and MinIO has met these needs. Also, latency has been reduced among the data centers; this can be expanded as needed while providing metadata for improved comprehension of such data. This also provides us very similar storage environments to the cloud platforms we are looking to move into in the near future.”
One of the cloud engineers noted similarly “MinIO has helped modernize our data analytics workloads, making them highly scalable, and has increased the adoption of cloud-native technologies within our organization.